
Recent attempts to achieve such robustness include domain classification based invariant detection, prior-knowledge based feature adaptation, adversarially-trained image alignment, map-specific domain adaptation, and physics-prior based zero-shot learning. Most object detection methods are designed for and trained with images captured under ideal environmental conditions, and often do not generalize to adverse settings (like foggy and low-light conditions). This requires robust perception which stems from object detection and semantic segmentation like tasks for recognizing and localizing safety-critical objects such as pedestrians and vehicles.
Gdip colorbot full#
READ FULL TEXT VIEW PDFĪutonomous mobile agents need a high-level understanding of their environment to plan their trajectories and function effectively. Synthetic datasets such as PascalVOC, and real-world foggy (RTTS) and State-of-the-art methods through quantitative and qualitative studies on Weĭemonstrate significant improvement in detection performance over several Resulting in higher throughput and plausible real-world deployment. Variant of GDIP that can be used as a regularizer for training Yolo, whichĮliminates the need for GDIP-based image enhancement during inference, Finally, trading off accuracy for speed, we propose a Weįurther improve GDIP through a multi-stage guidance procedure for progressive Outputs combined using weights learned through a novel gating mechanism. Image pre-processing (IP) techniques that operate concurrently, with their

This is achieved by learning parameters of multiple Proposed GDIP block learns to enhance images directly through the downstream We present a Gated Differentiable Image Processing (GDIP)īlock, a domain-agnostic network architecture, which can be plugged intoĮxisting object detection networks (e.g., Yolo) and trained end-to-end withĪdverse condition images such as those captured under fog and low lighting.
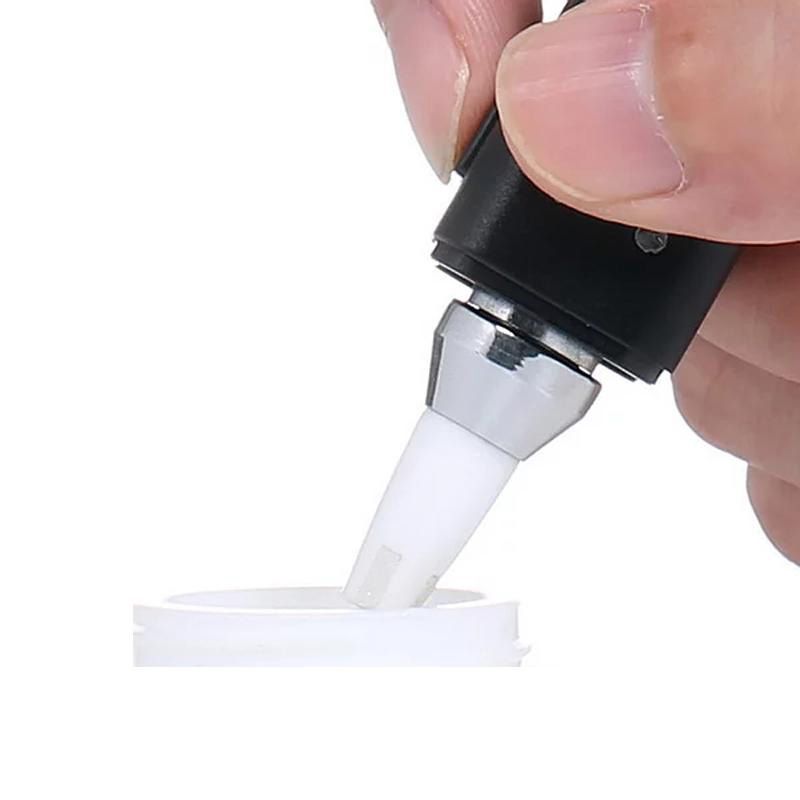
Detecting objects under adverse weather and lighting conditions is crucialįor the safe and continuous operation of an autonomous vehicle, and remains an
